Google Releases Hardware Accelerators for Machine Learning Apps
Google has released a new family of internally designed hardware
accelerators for speeding up certain machine learning workloads on the
company's cloud platform.
Google's new Cloud Tensor Processing Units (TPUs) are available for beta evaluation purposes starting this week. Each TPU comprises of four application specific integrated circuits (ASICs). A single TPU packs up to 180 teraflops of performance and up to 64 GB of high-bandwidth memory in a single board.
The boards can be used in standalone fashion or they can be linked together via dedicated network connections to form so-called TPU pods, which are basically multiple petaflop scale supercomputers for running machine learning apps, according to Google product managers John Barrus and Zak Stone. Google will offer the larger supercomputers to cloud platform customers starting later this year, the two product managers stated in a Feb. 12 blog.
Google's Cloud TPUs are designed to deliver improved cost performance for targeted machine learning workloads programmed with the TensorFlow open source software library, Barrus and Stone said. The technology will allow machine learning researchers and engineers to train, run and build their machine learning models more quickly than possible using current technology.
For instance, instead of having to wait for a shared computing resource to become available, machine-learning engineers can now get exclusive access to a dedicated Google Cloud TPU via a customizable Google Compute Engine virtual machine, the two engineers said.
Similarly, the new TPUs eliminate the need for machine learning researchers to spend days and even weeks in training a business critical model. "You can train several variants of the same model overnight on a fleet of Cloud TPUs and deploy the most accurate trained model in production the next day," according to Stone and Barrus.
Google has also made it possible for organizations to program the new Cloud TPUs without the highly specialized skills that are typically required when dealing with supercomputers and custom ASICs, they added. Google offers several high-level APIs for TensorFlow that organizations can use to get started right away.
Google also has released to open source a set of model implementations that companies can use as a reference for building programs that take advantage of the new Cloud TPUs. The reference models include those for image classification such as ResNet-50 and Densenet, one for object detection called RetinaNet and one for language modeling and machine translation.
"Cloud TPUs also simplify planning and managing [machine learning] computing resources," Barrus and Stone said. A cloud-hosted, tightly integrated, machine-learning computing cluster eliminates the need for organizations to maintain one on their premise. Such infrastructure can be costly for organizations to develop, deploy and maintain in-house.
A cloud-hosted infrastructure also gives enterprises the ability to scale up their requirements when needed and to scale it back down when it is no longer required, the Google product managers noted. (Via eWeek)
Google's new Cloud Tensor Processing Units (TPUs) are available for beta evaluation purposes starting this week. Each TPU comprises of four application specific integrated circuits (ASICs). A single TPU packs up to 180 teraflops of performance and up to 64 GB of high-bandwidth memory in a single board.
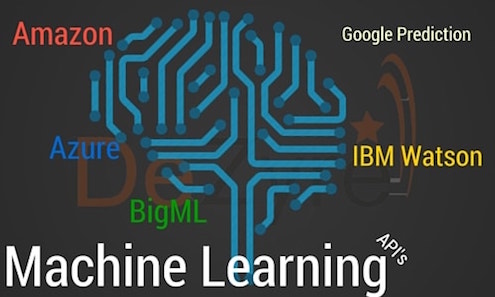
The boards can be used in standalone fashion or they can be linked together via dedicated network connections to form so-called TPU pods, which are basically multiple petaflop scale supercomputers for running machine learning apps, according to Google product managers John Barrus and Zak Stone. Google will offer the larger supercomputers to cloud platform customers starting later this year, the two product managers stated in a Feb. 12 blog.
Google's Cloud TPUs are designed to deliver improved cost performance for targeted machine learning workloads programmed with the TensorFlow open source software library, Barrus and Stone said. The technology will allow machine learning researchers and engineers to train, run and build their machine learning models more quickly than possible using current technology.
For instance, instead of having to wait for a shared computing resource to become available, machine-learning engineers can now get exclusive access to a dedicated Google Cloud TPU via a customizable Google Compute Engine virtual machine, the two engineers said.
Similarly, the new TPUs eliminate the need for machine learning researchers to spend days and even weeks in training a business critical model. "You can train several variants of the same model overnight on a fleet of Cloud TPUs and deploy the most accurate trained model in production the next day," according to Stone and Barrus.
Google has also made it possible for organizations to program the new Cloud TPUs without the highly specialized skills that are typically required when dealing with supercomputers and custom ASICs, they added. Google offers several high-level APIs for TensorFlow that organizations can use to get started right away.
Google also has released to open source a set of model implementations that companies can use as a reference for building programs that take advantage of the new Cloud TPUs. The reference models include those for image classification such as ResNet-50 and Densenet, one for object detection called RetinaNet and one for language modeling and machine translation.
"Cloud TPUs also simplify planning and managing [machine learning] computing resources," Barrus and Stone said. A cloud-hosted, tightly integrated, machine-learning computing cluster eliminates the need for organizations to maintain one on their premise. Such infrastructure can be costly for organizations to develop, deploy and maintain in-house.
A cloud-hosted infrastructure also gives enterprises the ability to scale up their requirements when needed and to scale it back down when it is no longer required, the Google product managers noted. (Via eWeek)
Post a Comment